AI tools are changing the publishing landscape and the value of human detection of scientific publishing fraud. This has given rise to a new kind of fraud buster. At STM innovations Day, held at the British Medical Association, London, in December 2023, Sabine Louët, founder of SciencePOD, had the opportunity to speak to one of the top experts in detecting problematic papers. His name is Cyril Labbé, and he is professor in computer science at the Grenoble Informatics Laboratory SIGMA team. He shares his views and experience in this interview.
LISTEN TO THE FULL INTERVIEW HERE
Detecting the fact that the text has been written by ChatGPT is, in itself, of no use. You really have to try to find out if the science behind what is written is good.
Cyril Labbé Tweet
Could you give us a top-level overview of the kinds of technologies that are available nowadays for publishers to detect fraud in scientific publications?
Well, it’s a difficult question because I think scientific literature is kind of under threat because more and more fake papers have been published. The publishing community needs to find a way to detect these fake papers more efficiently. And the ‘integrity services’ of the publishing community are not equipped enough to do this, they do not have enough people, they are not really managing to cope with the level of problems they encounter.
So that’s why they need to, and they are trying to, invest in new tools to detect these problematic papers.
Can you tell us about the types of technology that publishers typically use to detect such problematic papers?
Usually or traditionally, let’s say, the focus has been on trying to detect plagiarism. But more and more issues of concern are evident. There are problems with images, so they’re also trying to invest in new tools that will detect problematic images. You may know of Elizabeth Bik, for example, who is really skilled in detecting problematic images. So, they are trying to find tools that will do this kind of job, try to find out when an image in a scientific paper has been manipulated.
And then there are plenty of problems with text. Before ChatGPT, people were using paraphrasing tools to hide plagiarism. They were just asking an AI tool to rephrase text so that they could not be caught by a plagiarism detector, but these tools were making mistakes.
For example, the tools were using synonyms for each and every word. They might translate or paraphrase the words ‘artificial intelligence’ as ‘counterfeit consciousness’, for instance. And then you get this very strange wording and very incomprehensible text with completely tortured phrases, as we call them.
We can try to detect problematic text, problematic scientific papers by detecting these kinds of tortured phrases. Guillaume Cabanac and colleagues have created a tool that identifies suspect publications and builds a database of problematic papers.
We call it a problematic paper screener, it’s online and you can check it. You can find statistics there about where they were published. And you will see that all the big publishers have accepted, sold, and have taken APCs for these kinds of papers.
Tell us about ChatGPT. I imagine it has made your work even more interesting. What kind of clues are you looking for when you’re trying to detect articles written using generative AI?
ChatGPT has completely changed the landscape. Now, the paper mills, the companies that specialise in generating papers aimed at scientists, they can use ChatGPT as a boost for productivity. So, we need to detect these kinds of papers that are generated using ChatGPT.
But the problem is that ChatGPT can also be used in a very ethical way, in a very good way. You can use Chat GPT to re-write a sentence in correct English that may have been badly written originally. That’s perfectly fine; this is a good use of Chat GPT. It could be very useful for me [as a non-native English speaker], for example, to make my English much better.
So, detecting the fact that the text has been written by ChatGPT is, in itself, of no use. You really have to try to find out if the science behind what is written is good. What we need to know is if there is a hallucination, or fake content, or something that should have been done or reported but was not, etc. These are the kinds of things that we should try to find.
But for now, the only thing that we are able to detect is obvious errors. Like for example, the ‘regenerate’ button that has been copy-pasted and inserted in the text. You can find in some scientific papers the ‘regenerate response’ in the middle of a sentence, or a response indicating that the model language cannot generate a response, or a response or sentence that is cut off, something like that. These are clues that ChatGPT was used. But then you have to check – a human has to check – If the content is right or not.
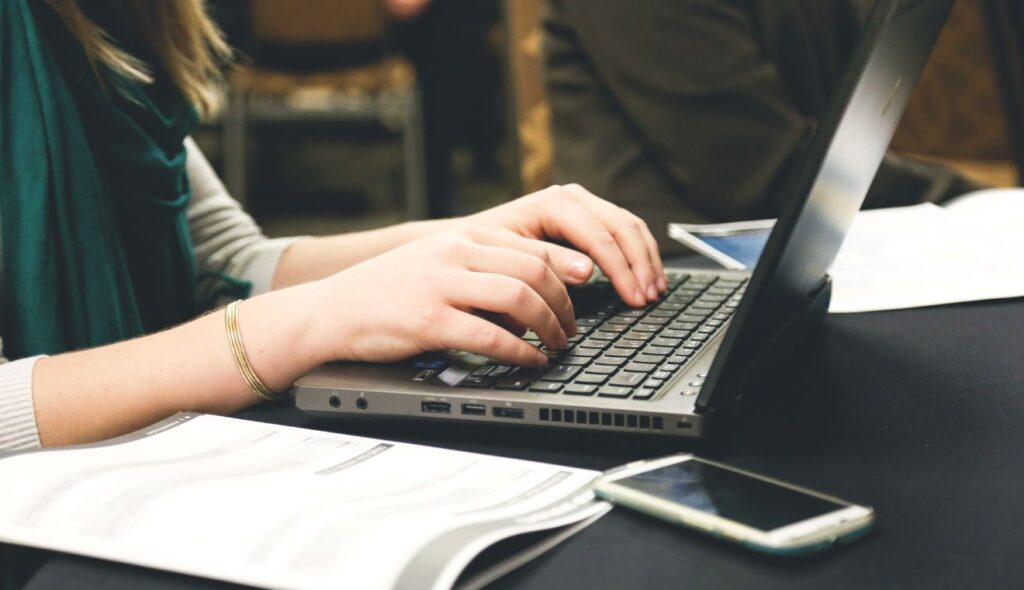
Is there a kind of archive or catalogue of all these erroneous language fingerprints for problematic papers?
Yes, so we call them fingerprints and we have a list that is available online. This list has been built by many people because there is a community behind this. A lot of people are trying to find all these strange papers, and they are posting comments on PubPeer, which is an open platform for people to express and to post comments on different scientific paper.
So, there are plenty of people looking out for new fingerprints that we didn’t know about before. This list has been built through a kind of snowball effect, with plenty of people participating in the creation of the list.
Where can we find this list?
The list is available on the problematic paper screener, site, plenty of people are using it, in fact, to integrate into their own tools. For example, the STM Integrity Hub is using this list to detect tortured phrases or text that has been badly paraphrased
How much does the publishing industry call upon your expertise nowadays? Do you have collaborations with individual publishers or with learned societies and research associations?
We are collaborating with anyone that wants to collaborate with us, and we do it entirely pro bono. We have not accepted funding from the different publishers that we help. We work with IOP, for example, which is at the forefront of addressing these integrity problems. We work with STM Integrity Hub, with Morressier. We collaborate with anyone who asks us ‘where is this list?’ We provide the list for them to use. It’s completely free, and available to anybody.
Where do you see next generation tools coming from? And what kind of angle do you anticipate will be used from a technology perspective to detect suspect publications?
I think there is a new threat coming, beyond the detection problem – that is the fact that new models are able to generate data as well as text.
These new multimodal models are able to do many things, such as generate graphs together with text and images, etc. These are really useful tools for people who want to generate scientific papers just by pressing a button. So yes, there is a problem here. And the way to detect this problem, I believe, is to go beyond simply detecting whether a publication has been generated or not.
Instead, we need to try to detect the scientific meaning behind the text, and to check that there is really something behind what is published. We need to take the ‘open science’ approach, supporting the release of data so that people can check and assess what is in a text.
And I think that publishers have to invest in human labour. They need to ensure that peer review is done correctly and invest in post-publication assessment. They should be able to deal with retractions and corrections and things like that much more easily and speedily.
What you’re saying is that the technology can only do so much and then the rest of the work has to be done by skilled humans who understand where the flaws could come from?
Yes, that’s my take, that’s where I stand on this.