This article delves into the complex realm of algorithmic bias in artificial intelligence (AI), a phenomenon that can inadvertently perpetuate societal prejudices.
We will explore how this bias occurs, its far-reaching implications, and methods for mitigation.
Understanding and addressing algorithmic bias is not just a technical necessity, but a moral imperative, to ensure fairness and equality in AI applications across sectors like healthcare, finance, and criminal justice.
Key Takeaways
- Algorithmic bias refers to systematic and repeatable errors in computer systems that result in unfair outcomes.
- Algorithmic bias can lead to inadvertent privacy violations and reinforce social biases related to race, gender, sexuality, and ethnicity.
- Algorithmic bias can arise from pre-existing cultural, social, or institutional expectations, as well as technical limitations in algorithm design.
- Algorithmic bias has been observed in various domains, including criminal justice, healthcare, and hiring.
Comprehending the Definition and Impact of Algorithmic Bias
The ongoing discussion on comprehending the definition and impact of algorithmic bias underscores its significance, particularly its capacity to result in systematic errors in computer systems that can lead to unfair outcomes, potentially exacerbating disparities in various sectors such as healthcare, finance, and criminal justice.
Algorithmic bias may arise when a computer system, trained on biased data or under biased conditions, begins to exhibit those same biases in its operations. For instance, a hiring algorithm trained mostly on successful applications from a certain gender could inadvertently discriminate against the other.
This is what algorithmic bias is, and these are just a few examples of algorithmic bias.
It is crucial to understand and rectify this issue to ensure fairness and equality in the digital age.
Unraveling the Causes and Challenges in Detecting Algorithmic Bias
In our ongoing discourse, we are shifting focus towards the causes and challenges inherent in detecting algorithmic bias, a complex issue that requires careful analysis and comprehensive understanding of both the design and deployment of algorithms, and the data upon which they are trained.
One primary cause of algorithmic bias is the use of skewed or unrepresentative training data, which can inadvertently favour certain demographics over others.
Furthermore, the proprietary nature of many algorithms, coupled with their inherent complexity, poses significant obstacles to understanding and detecting bias. Even when full transparency is provided, the multifaceted nature of algorithms can make it difficult to pinpoint where bias originates.
This, combined with the relative inability to access proprietary datasets, compounds the challenge of effectively diagnosing and addressing algorithmic bias.
A Historical Overview and Real-world Instances of Algorithmic Bias
Drawing from historical perspectives and real-world examples, our exploration of algorithmic bias now delves into its evolution over time and its manifestation in contemporary systems, revealing both the tenacity and ubiquity of this complex issue. The origins of algorithmic bias are intertwined with the initial development of computer systems and have manifested in various sectors such as healthcare, finance, and recruitment.
Historical Period | Manifestation of Algorithmic Bias |
---|---|
1960s-1970s | Mainframe computers exhibiting bias |
1980s-1990s | Biased algorithms in hiring practices |
2000s | Social media algorithms amplifying misinformation |
2010s | Facial recognition systems misidentifying individuals |
2020s | Biased AI in healthcare leading to unequal treatment |
These instances underline the persistent challenge of mitigating algorithmic bias, highlighting the need for continuous vigilance, robust legislation, and innovative technological solutions.
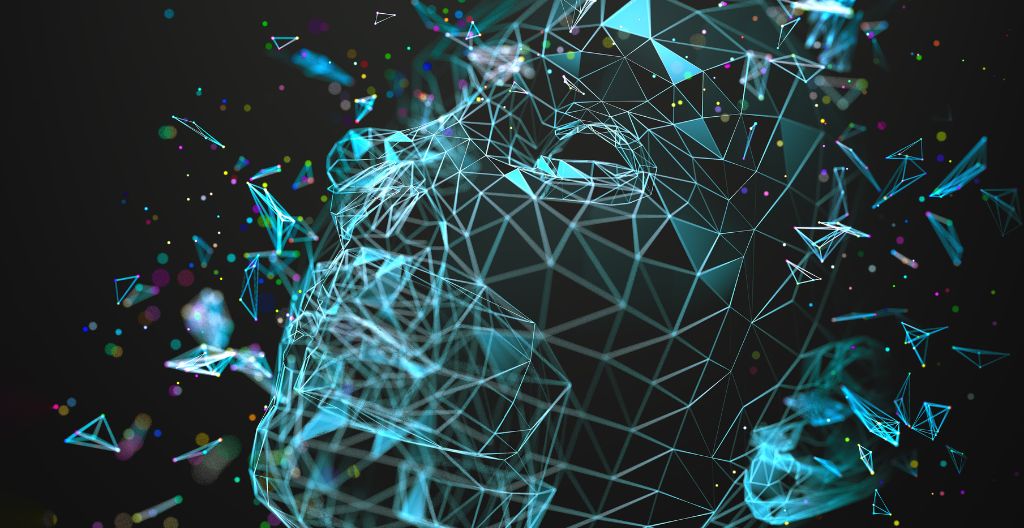
Dissecting Different Types and Manifestations of Algorithmic Bias
While exploring the intricate landscape of algorithmic bias, we must consider both its diverse types, such as pre-existing bias and technical bias, and its varied manifestations across sectors, from healthcare to criminal justice.
Pre-existing bias stems from socially ingrained prejudices that infect datasets, leading to unjust outcomes. Technical bias, on the other hand, arises from limitations within the algorithmic design process itself.
These biases can manifest in myriad ways. For example, in healthcare, skewed data can lead to misdiagnosis or inadequate treatment suggestions. In the criminal justice system, biased algorithms have been known to disproportionately target certain racial groups.
Hence, understanding these biases is crucial to prevent the propagation of unfair practices and to ensure equitable outcomes in the era of digital decision-making.
Strategies and Techniques for Mitigating Algorithmic Bias
We need to delve into effective strategies and techniques that can be employed to mitigate the pervasive issue of algorithmic bias in various sectors.
Firstly, bias detection and mitigation tools should be incorporated in the algorithm development process.
Secondly, the diversity within development teams needs to be increased to prevent unconscious biases from influencing algorithm design.
Thirdly, transparency in algorithm functioning and decision-making can foster accountability.
Fourthly, the use of unbiased, representative, and updated datasets for training AI models is crucial.
Lastly, legislation must be put in place to regulate algorithmic decision-making and to penalise biased outcomes.
Sign up for our Life Sciences Newsletter and boost your engagement with HCPs
Frequently Asked Questions
How Does a Technical Content Agency Address Algorithmic Bias in Content Development?
A technical content agency plays a vital role in addressing algorithmic bias by implementing rigorous content review processes and guidelines. They ensure that technical content is free from biases and adheres to ethical standards, helping to mitigate the risk of bias in algorithms and technology systems that rely on their content.
How Does Algorithmic Bias Affect the Field of Education, Particularly in Student Evaluation and Learning Analytics?
Algorithmic bias in education can lead to unfair student evaluations and skewed learning analytics, potentially disadvantaging certain groups of students and reinforcing existing educational disparities based on data-driven decision-making processes.
Can Artificial Intelligence Be Developed to Detect and Correct Its Own Algorithmic Bias?
Yes, artificial intelligence can be developed to detect and correct its own algorithmic bias. This involves creating self-auditing algorithms, using diverse datasets, and employing fairness metrics in the AI development and validation process.
What Are Some Real-World Consequences of Algorithmic Bias in the Entertainment and Media Industry?
Algorithmic bias in the entertainment and media industry can lead to skewed content recommendation, promoting a lack of diversity in viewed content, reinforcing stereotypes, and limiting exposure to different perspectives and cultures.
How Does Algorithmic Bias Interplay With Other Technological Biases Such as Design Bias and Data Bias?
Algorithmic bias interplays with design and data bias as flawed design can introduce systematic errors, while biased data can skew outcomes. Both can compound algorithmic bias, leading to more unfair and discriminatory results.
What Are Some Emerging Trends or Technological Advancements AImed at Preventing Algorithmic Bias in AI Development?
Emerging trends aimed at preventing algorithmic bias include employing diverse development teams, enhancing transparency of AI systems, implementing bias audits, and utilising fair machine learning techniques to ensure unbiased data training and algorithm development.
How Does User Generated Content Contribute to Algorithmic Bias?
User generated content can inadvertently contribute to algorithmic bias when it reflects or amplifies existing biases, stereotypes, or prejudices held by individuals within the user base. Algorithms that rely on such content may then perpetuate and amplify these biases, leading to unequal or unfair outcomes in various online systems and platforms.
How Can Technical Writing Mitigate Algorithmic Bias in Content and Documentation?
Technical writing plays a critical role in addressing algorithmic bias by ensuring that documentation and instructions are clear, unbiased, and inclusive. By implementing best practices in technical writing, content creators can help reduce the potential for bias in algorithms and promote fairness and equity in technology.
Conclusion
In conclusion, algorithmic bias in AI presents a significant challenge, affecting numerous sectors and impacting individuals and groups. Understanding its causes and manifestations is crucial for developing effective mitigation strategies.
By ensuring ethical AI practices, it is possible to reduce the negative impacts of algorithmic bias and enhance fairness in AI-driven decision-making, thereby contributing to a more equitable digital age.
It remains essential to continue the discourse on this critical issue and encourage responsible AI usage and development.